
Executive Summary
Artificial Intelligence (AI) holds enormous potential, promising transformative benefits across industries. However, this potential can quickly turn into a costly liability if not grounded in high-quality data. The historical lessons from the 2007-2009 financial crisis highlight the catastrophic risks of relying on flawed data, a warning that is even more pertinent in the age of AI. For companies eager to harness AI’s power, ensuring the accuracy, relevance, and integrity of their data is not merely a technical requirement—it’s a strategic imperative. This article provides detailed insights into the essential steps leaders must take to manage data quality effectively, thus safeguarding their AI initiatives from failure.
Introduction: The Cost of Neglecting Data Quality
In the rapidly evolving landscape of AI, data is often referred to as the new oil—an essential resource driving innovation and competitive advantage. However, just as crude oil must be refined before it can power engines, data must be meticulously curated and verified to fuel AI models effectively. Neglecting this critical step can lead to AI models that produce inaccurate, biased, or even harmful outcomes. The parallels between today’s AI-driven enterprises and the financial institutions of the late 2000s, which suffered massive losses due to poor data quality, are stark. By examining the failures of the past, we can understand why ensuring data quality must be a top priority for any organization venturing into AI.
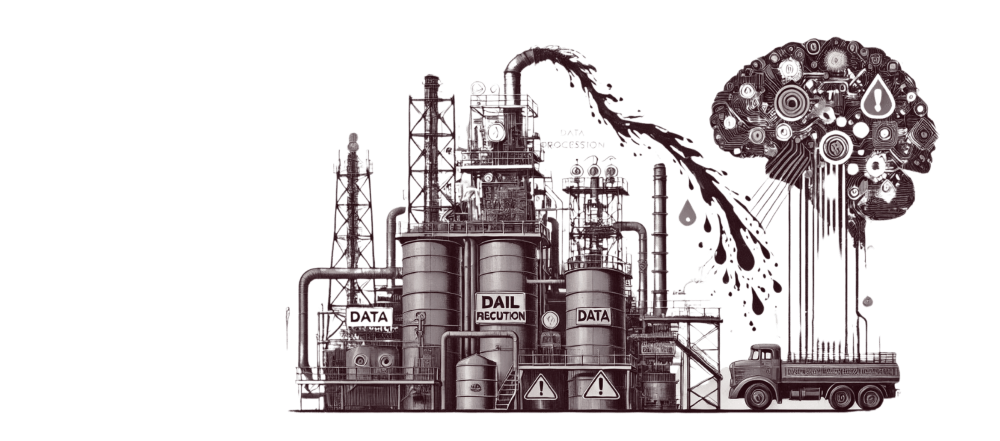
Lessons from the Past: Data Quality and Business Risk
A Cautionary Tale: The 2007-2009 Financial Crisis
The 2007-2009 financial crisis serves as a powerful reminder of the dangers of poor data quality. Financial institutions heavily relied on complex financial instruments like mortgage-backed securities (MBS) and collateralized debt obligations (CDOs), which were underpinned by data that was, at best, incomplete and, at worst, fraudulent. The risk models used to price these securities assumed that the underlying data was accurate, leading to a gross underestimation of risk. When the reality of widespread foreclosures set in, the entire financial system was brought to its knees. The lesson is clear: even the most sophisticated analytics cannot compensate for bad data.
Why AI is Even Riskier
While the financial crisis was devastating, the risks associated with AI are even more profound. The mathematics used to create MBS and CDOs were well understood by financial experts, but AI operates in a much more complex and opaque domain. AI models are often referred to as “black boxes” because even experts struggle to understand exactly how they arrive at their predictions. This lack of transparency, combined with the vast amounts of data required to train AI models, makes the quality of that data even more critical. If the data is flawed, the consequences can be far-reaching, affecting individuals, companies, and entire societies.
Moreover, the accessibility of AI tools has lowered the barrier to entry, allowing individuals and organizations with little understanding of data quality to deploy AI models. This democratization of AI increases the risk that flawed models will be used in high-stakes decisions, leading to potentially disastrous outcomes.
Understanding the Problem: Aligning Data with Business Goals
Start with the Right Questions
Any successful AI initiative must begin with a clear and precise understanding of the problem it seeks to solve. For instance, consider a financial institution aiming to improve its loan approval process. The initial problem statement might be, “We need a better way to decide which loans to grant and under what terms.” However, this statement is just the starting point. To align the AI model with business goals, the organization must ask more specific questions:
- “Better how?” Does “better” mean reducing the default rate, minimizing bias, or increasing profitability?
- “Which loans to whom?” Should the model focus on specific demographics, geographical regions, or types of loans?
The answers to these questions will determine the type of data required. For example, if “better” means reducing bias, the training data must be free from historical biases, which may be challenging to achieve. If the goal is to increase profitability, the data should include predictive indicators of loan performance, which might require incorporating data from loans that were previously rejected.
Avoid the Common Pitfalls
Defining the problem is often a politically charged process. Different stakeholders may have conflicting interests. For example, those eager to bring AI into the organization may want to demonstrate its effectiveness quickly, while others may be more concerned with regulatory compliance or mitigating competitive risks. Moving forward without resolving these conflicts can lead to significant challenges later in the project. A poorly defined problem can result in selecting inappropriate data, leading to an AI model that fails to deliver the expected results.
Warnings about data quality have been plentiful, coming from diverse sources leading the AI revolution, including Google, Meta, Open AI; prominent researchers; and the popular press including the New York Times and the Economist. Forrester, the research firm, advises that “Data Quality Is Now the Primary Factor Limiting Gen AI Adoption.” Yet most companies have not even started the work they need to do.
Getting Data Right: A Two-Pronged Approach
1. The “Right Data”
Identifying the “right data” is a crucial step that goes beyond simply having a large dataset. The data must be relevant to the problem at hand, comprehensive in its coverage, and free from bias. This involves ensuring that the data adequately represents all populations or scenarios the AI model will encounter. For example, if an AI model is intended to work globally, the training data must include inputs from all relevant geographical regions to avoid skewed predictions.
- Relevance and Completeness: AI models require data that has predictive power. For loan approval processes, relevant attributes might include payment history, income, and credit scores. Completeness is equally important—missing attributes can lead to inaccurate predictions.
- Comprehensiveness and Representation: The data must cover the entire population of interest, as well as all relevant subpopulations. Without adequate representation, the AI model may perform well in some scenarios but fail in others, leading to biased or incomplete results.
- Freedom from Bias: Historical data often reflects the biases of the time it was collected, which can be problematic when training AI models. If bias cannot be removed from the data, it may be better to reconsider using AI for that particular application.
- Timeliness: The data used to train AI models must be current. In fast-moving industries like finance, even small delays in data can lead to significant errors in AI predictions.
- Clear Definition: Data often comes from multiple sources, each with its own definitions and standards. Ensuring that data is clearly defined and consistently measured across sources is critical for integrating it into a cohesive dataset.
- Appropriate Exclusions: Certain data points, such as zip codes in loan decisions, can act as proxies for sensitive information like race. Excluding these data points is essential to avoid legal and ethical issues, as well as to ensure the AI model complies with privacy regulations.
2. Data Integrity
Even with the right data, the integrity of that data must be maintained. This includes ensuring accuracy, consistency, and correct labeling.
- Accuracy: Data accuracy is the foundation of any successful AI model. Inaccurate data can lead to incorrect predictions and ultimately, poor business decisions. For example, in the loan approval process, inaccurate credit scores can result in either denying creditworthy applicants or approving high-risk ones.
- Absence of Duplicates: Duplicate entries can skew AI model outputs, leading to overrepresented or misrepresented data. It’s crucial to identify and eliminate duplicates to ensure the dataset accurately reflects the population.
- Consistent Identifiers: Merging data from different sources requires consistent identifiers to match records accurately. For example, ensuring that “John Smith” in one database is the same individual as “J. E. Smith” in another is essential for integrating data correctly.
- Correct Labeling: Proper labeling of data is critical for training AI models. Incorrect labels can lead to flawed models that produce unreliable results. For instance, if a model is trained on mislabeled loan outcomes, it may fail to predict defaults accurately.
Short-Term Strategy: Adopt a “Guilty Until Proven Innocent” Approach
Management’s Role
In the short term, organizations must take a proactive approach to data quality by adopting a “guilty until proven innocent” stance. This means assuming that data is flawed until it has been thoroughly vetted. Senior management should take ownership of data quality, ensuring that it is prioritized across the organization and not left solely to technical teams.
- Assigning Responsibility: Data quality management should be the responsibility of the highest-level person overseeing the AI project. This individual must assemble teams to develop quality requirements and ensure they are met.
- Relentless Questioning: Managers should relentlessly question data sources, training data criteria, and the steps taken to clean and integrate data. This includes asking how accuracy was measured, how duplicates were handled, and how data labels were verified.
- Vendor Caution: When working with vendors, it’s important to ask detailed questions about the data they provide. Be wary of vendors who refuse to disclose information, citing proprietary models. While the model may be proprietary, the data should not be.
Vigilance Over Future Data
Training an AI model on high-quality data is just the first step. The model’s performance will degrade if it is later fed poor-quality data. Managers must monitor future data closely to ensure it remains consistent with the training data. This requires rigorous controls to maintain data quality over time, preventing the model from producing inaccurate or biased results.
- Continuous Monitoring: Implementing in-depth controls and regular audits to ensure future data aligns with the quality of the training data is essential. This includes checking for changes in data inputs that could affect model performance.
- Independent Review: Senior leaders and boards should insist on independent quality assurance reviews to verify that all aspects of data quality are being properly managed. Early in the AI adoption process, it is better to have too much oversight than too little.
Mid-Term Strategy: Shift Focus Upstream
Eliminate Data Problems at the Source
In the mid-term, organizations should shift their focus from fixing data problems downstream to preventing them upstream. This involves identifying and eliminating the root causes of bad data, thereby reducing the amount of time spent on data cleanup and allowing data scientists to focus on higher-value tasks.
- Data as a Product: Treating data as a product involves setting clear quality standards and ensuring that all teams within the organization adhere to them. This includes establishing roles where downstream teams act as “data customers” and upstream teams as “data creators,” working together to meet quality requirements.
- Collaboration and Accountability: Encouraging collaboration between teams and holding them accountable for the quality of the data they produce can lead to significant improvements in data integrity. By addressing errors at their source, companies can reduce the amount of time spent on data cleanup and improve the overall quality of their AI models.
Customer and Process-Oriented Data Management
By treating data as a product and fostering collaboration between teams, organizations can create a culture of continuous improvement. This involves setting clear expectations for data quality and regularly reviewing processes to identify areas for improvement.
- Focus on Customer Needs: By understanding the needs of the downstream teams (the data customers), upstream teams (the data creators) can better align their processes to produce high-quality data that meets those needs.
- Continuous Improvement: Implementing a process-oriented approach to data management encourages continuous improvement, ensuring that data quality issues are identified and addressed before they become significant problems.
Long-Term Vision: Transforming Data Management
Invest in Data Quality
In the long term, investing in data quality upfront can save costs and prevent the need for costly corrections later. Organizations should view data quality management as a strategic initiative that supports their overall business goals.
- Cost Savings: High-quality data reduces the time and resources needed for data cleanup, allowing data scientists to focus on more valuable tasks. This can lead to significant cost savings and improved efficiency.
- Strategic Advantage: By ensuring high data quality, organizations can gain a competitive edge, using AI to drive innovation and improve decision-making.
Leadership and Culture
Building a data-driven culture requires commitment from the top. Leaders must champion data quality initiatives, ensuring that accurate and relevant data is prioritized throughout the organization. This involves setting clear expectations, providing the necessary resources, and fostering a culture that values data quality.
- Leadership Commitment: Senior leaders must take an active role in promoting data quality, ensuring that it is embedded in the organization’s culture and practices.
- Organizational Culture: Fostering a culture that values data quality involves educating employees about the importance of accurate and relevant data, encouraging collaboration, and rewarding those who contribute to improving data quality.
Conclusion: The Path to High-Quality AI
High-quality AI is built on high-quality data. Organizations that invest in data quality from the start are better positioned to harness AI’s full potential, driving innovation and growth while minimizing risks. The journey towards better data quality is challenging, but it is essential for any organization serious about leveraging AI to gain a competitive advantage. By prioritizing data quality, organizations can ensure that their AI initiatives deliver reliable, ethical, and impactful results.
Article inspired by Ensure High-Quality Data Powers Your AI, written by Thomas C. Redman